A dose for a healthier business
Data governance in small and medium pharmaceutical enterprises
Authors: Katherine Lewis, Dr. Anh Mattick, Andrea Wuchner
Healthcare enterprises can be described as being very data rich but sometimes information poor due to a lack of organization and intentional direction with regards to data management. The personal health data that they handle represents some of the most sensitive and confidential types of data to be responsible for, and therefore a greater onus is placed on healthcare providers and industries such as biomedical or pharmaceutical companies to protect and safeguard privacy.
Failure to implement appropriate data governance may result in data breaches, loss of public trust, and fines or penalties from regulatory actors [1]. The implementation of a data governance framework will ensure that an organization operates within the boundaries of safety and facilitate the development of innovative business models and the achievement of broader organizational goals [2].
Data governance is the comprehensive process of defining the infrastructure of an organization’s data through a framework that outlines the routine actions to be taken with the data and the individuals within the organization who have access to it and are responsible for its stewardship. The Data Management Book of Knowledge (DMBoK) published by DAMA International defines data governance as »the exercise of authority, control, and shared decision-making (planning, monitoring, and enforcement) over the management of data assets« [3].
The terms data governance and data management are often conflated. Data governance is the exercise of authority, control, and shared decision-making (planning, monitoring, and enforcement) over the management of data assets. Data management, in contrast, is the actual implementation and continual maintenance of data in accordance with policies and guidelines established by a framework [4].
A framework is designed to ensure the effective management and utilization of data as a resource. In essence, data governance establishes the foundation for the management of data in a manner that maximizes its value as a resource for an organization to utilize, while minimizing the potential for associated risks that may harm the organization. Without proper data governance, precious data may metaphorically ‘fall through the cracks’ within an organization and go unused, or worse, misused. Data assets represent a valuable resource for any organization. The implementation of a data governance framework ensures that data is optimized to its full potential [5].
Pharmaceutical companies face unique demands for appropriate data governance due to the nature of the information they collect. This includes sensitive and confidential patient and healthcare information, in addition to the usual data that any company generates and collects, such as marketing and sales, product research and development, and so on (see Figure 1).
Furthermore, pharmaceutical companies are expected to adhere to good practice standards in their laboratories and clinics, generating what is known as GxP data, which is crucial for maintaining adherence to standards required by various regulatory agencies [2].
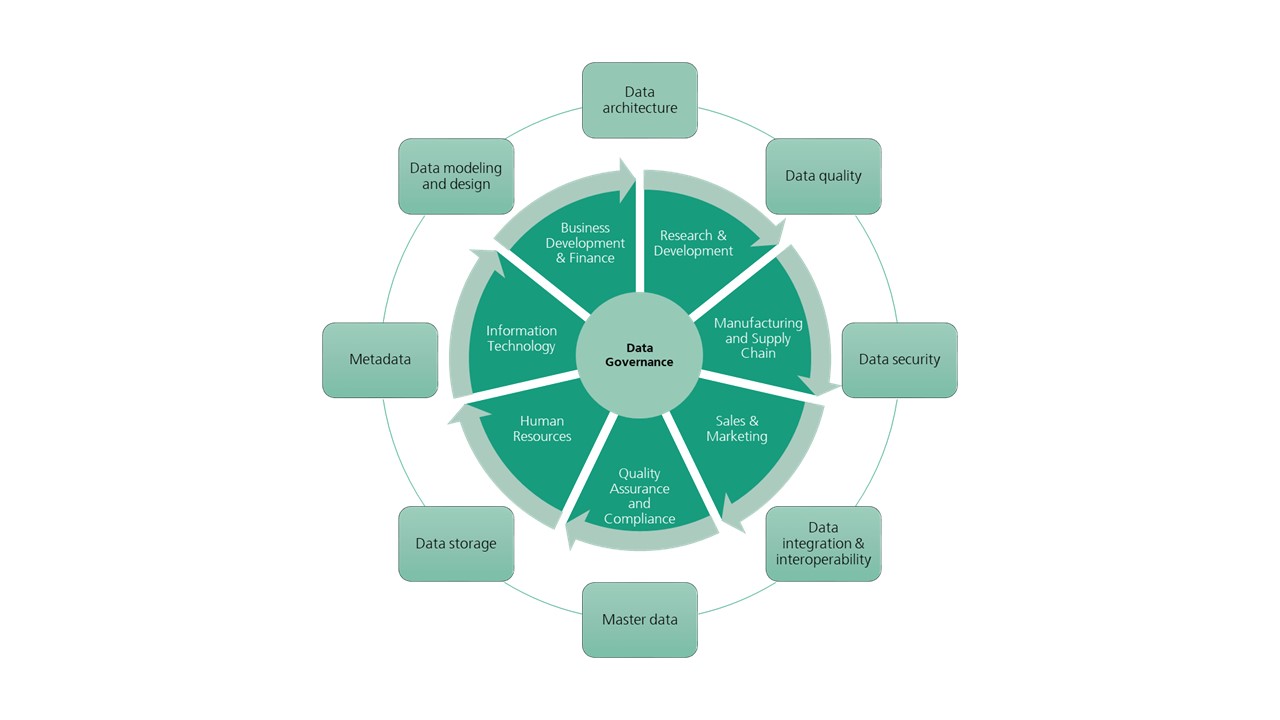
One advantage of implementing a data governance framework is that it facilitates the transfer and accessibility of data to the appropriate personnel within an organization. Pharmaceutical companies are comprised of numerous departments that are, while distinct, interdependent. Laboratory development, clinical research, sales, finance and business development, and manufacturing are some examples. The proper governance of an organization’s central data repository ensures that departments are not inundated with access to data that they will never require, and that they have immediate and automated access to the data that they do require.
For instance, the customer service department will have streamlined access to relevant data collected by the sales department, such as contact information or sales history of a partnering healthcare provider. This facilitates more efficient communication between the company and the consumer and eliminates the possibility of multiple intakes of the same data every time a consumer deals with a different department. Furthermore, the implementation of data governance policies ensures that employees engaged in the commercialization of a product are unable to access any confidential proprietary developmental data or clinical data containing the health information of trial patients.
The advent of new technologies and the emergence of digital health tools, such as medical wearable devices and mobile phone monitoring sensors, has led to the generation of a vast array of data assets. Pharmaceutical companies have already begun utilizing data collected from such wearable devices to track heart rate, physical activity, sleep patterns, and other parameters, for the purpose of clinical research and the development of new drugs and treatments. Now, data that was previously difficult, costly, and sometimes inaccurate to collect is reliable and convenient for researchers and clinicians to use [6]. Prioritizing improved data governance and establishing standards and protocols for data collection across diverse sources will assist companies in maintaining data uniformity and integrity, and allow seamless integration whenever novel sources are considered. Wearable health monitoring devices have been embraced by the general population for almost a decade, and the biomedical technology and engineering industry continues to develop new methods for harmlessly collecting health data. Companies have numerous incentives to act promptly in order to maintain a competitive edge in the market.
The demand for the implementation of data governance frameworks is particularly pronounced when they are tailored to the specific needs of small and medium-sized pharmaceutical enterprises (Pharma SMEs). Firstly, pharmaceutical SMEs are more likely to be in the nascent stages of their development and focused on improving their business models to accommodate growth [7]. The longer a company delays the implementation of data governance, the more challenging it will be to integrate and make decisions, given that the volume of data continues to grow with each passing day that the company operates [8]. Secondly, pharma SMEs play a significant role in the research and development of new drugs [9]. Larger pharmaceutical companies frequently depend on their »blockbuster« patented drugs to maintain profits. They also seek out small and medium-sized enterprises (SMEs) with new ideas and focused expertise to remain at the forefront of the market. This is because it is more cost-effective for them to collaborate with SMEs than to create new R&D teams within their own enterprise. Acquisitions and partnerships with larger pharmaceutical companies represent a significant source of financing for SMEs engaged in clinical research and new product development [10]. This symbiotic strategy involves adopting a data governance framework in that it is one tool pharma SMEs have available to successfully grow their business. This growth enables them to attract the attention of potential partners who have the opportunity to view the full catalogue of data assets and to appreciate the ways in which the data has been optimized as a resource. A key obstacle identified for SMEs of all industries in regard to data governance is that they often, »fundamentally lack the scale, the scope, the necessary capital, and the personnel (data scientists, data engineers, etc.)« needed for building a customized framework that matches the goals and desired business model of the company [11].
We suggest a multi-layered competence-based data governance approach that offers a solution to these challenges (see Figure 2). Such a data governance approach ensures a systematic integration of the organization, its people and its technology. At the organizational level, it is necessary to develop customized strategies and frameworks that meet the specific needs and objectives of the organization. At this stage, it is critical to establish a clear data governance vision and strategy that is aligned with the organization‘s overall business strategy, and to establish a data governance council or steering committee to oversee the implementation and enforcement of data governance policies. At the people level, employees need to be optimally prepared and skilled with defined policies and processes and clearly defined data management responsibilities and roles. Data governance encapsulates cultural and philosophical organizational change that requires long-term commitment to doing business differently [8]. Therefore, for data governance to be effective, the chosen approach must involve the relevant actors and roles must be defined, as data governance is not a product that can be bought, implemented, and left within an organization‘s infrastructure.
To this end, it requires a competency-based approach that prepares employees for their respective roles, functions and associated competencies and strengthens their skillset. Centering data governance transformation around the process of skills training works off the logic of the classic proverb “If you give a man a fish, you feed him for a day. If you teach a man to fish, he will eat for a lifetime.” There is a documented need for competence-learning based solutions. Research conducted with Singaporean SMEs yielded that 50% of respondents stated they lack the necessary in-house expertise and skills to successfully adopt new innovations within their business To this end, it requires a competency-based approach that prepares employees for their respective roles, functions and associated competencies and strengthen their skillset To this end, it requires a competency-based approach that prepares employees for their respective roles, functions and associated competencies and strengthen their skillset—effectively stagnating their growth and competitiveness [7, 12].
At the technology level, it is necessary to select and integrate technologies and tools to improve data security, quality and usage, and ensure that organizations meet legal and regulatory requirements. A robust data architecture that supports the organization’s data governance objectives, including data integration, storage, and processing capabilities ensures sustainable implementation. Metadata management is a relevant aspect which entails utilizing metadata management tools to ensure proper documentation and understanding of data assets and implementing a centralized metadata repository to provide a single source of truth for data definitions, lineage, and usage.
Data governance is a transformative change process that only takes place if all key stakeholders are involved and the infrastructure is in place to carry out the process. Our approach helps organizations to implement efficient data governance that integrates the organization as a whole with its employees and its technological infrastructure on the path to transformation.
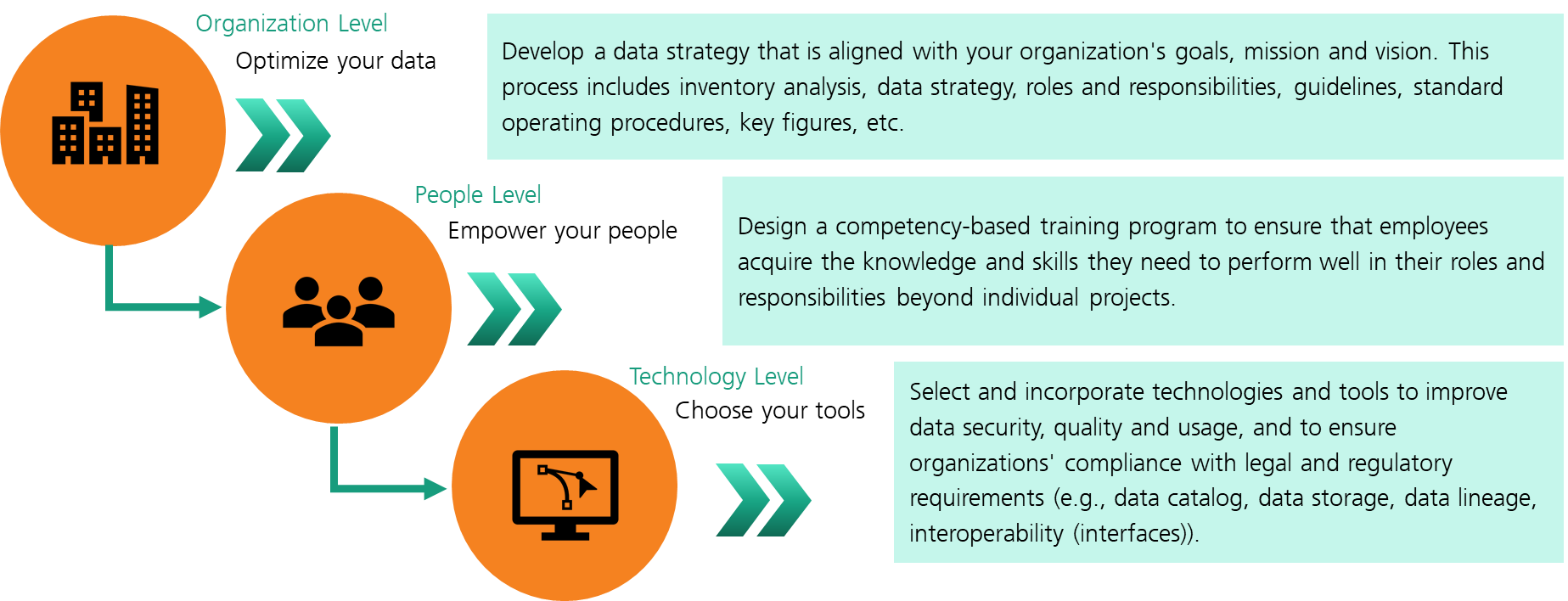
In conclusion, the current body of literature provides sufficient evidence of the pivotal role that data governance plays in healthcare industries. This role is evident in the assurance of data integrity, the facilitation of informed decision-making, the adherence to compliance regulations, the monitoring of product quality and performance, and the establishment of a robust foundation for the introduction of new forms of data in the event of technological developments.
Focusing on small and medium pharmaceutical companies, the current literature indicates that investing in an appropriate data governance framework can accomplish such goals, while also providing opportunities to innovate business models in agreement with a company’s goals whether they may be optimizing efficiency, better fueling R&D, providing new information to marketing, attracting larger corporate partnerships, and beyond. This article presents a brief overview of the incentives pharma SMEs have towards implementing effective data governance, and the options available to them in the face of challenges inherent to a SME, such as a lack of data governance-competent personnel.
We propose an integration of organizational, workforce and technology perspectives in data governance to align the policies, processes and tools used for data management with the objectives, responsibilities and capabilities of all stakeholders. Further research in this area, including interviews with experts in the pharmaceutical and biotechnology industries, may yield insights into additional incentives and obstacles that can be incorporated into the existing literature. The impact of SMEs on global economies cannot be overstated, with their output representing 50% of GDP in high-income countries worldwide [12].
Further investigation into the potential for governments in high-income countries to provide support to SMEs through the challenges they face would also be beneficial.
References
[1] H.-G. Eichler, B. Bloechl-Daum, K. Broich, et al., »Data Rich, Information Poor: Can We Use Electronic Health Records to Create a Learning Healthcare System for Pharmaceuticals?« Clinical Pharmacology & Therapeutics, pp. 912–922, October 2018.
[2] R. McDowall, »Understanding data governance, part i,« Spectroscopy, pp. 32–38, February 2017.
[3] Dama International, DAMA-DMBOK: Data Management Body of Knowledge, 2nd ed., Technics Publications, 2017.
[4] V. Khatri and C. V. Brown, »Designing Data Governance,« Communications of the ACM, pp. 148–152, January 2010.
[5] A. Al-Badi, A. Tarhini and A. I. Khan, »Exploring Big Data Governance Frameworks,« in EUSPN, 2018.
[6] M. Rogozinska, »Can the Use of Novel Digital Devices Improve the Productivity of Drug Development?« Clinical Pharmacology and Therapeutics, pp. 35–37, July 2018.
[7] D.-Y. Lin, S. N. Rayavarapu, K. Tadjeddine and R. Yeoh, »Beyond financials: Helping small and medium-size enterprises thrive,« McKinsey & Company, 2022.
[8] J. Ladley, Data Governance: How to Design, Deploy, and Sustain an Effective Data Governance Program, Academic Press, 2019.
[9] C. Årdal, E. Baraldi, U. Theuretzbacher, et al., »Insights into early stage of antibiotic development in small- and medium-sized enterprises: a survey of targets, costs, and durations,« Journal of Pharmaceutical Policy and Practice, April 2018.
[10] E. Madsen and Y. Wu, »Low R&D Efficiency in Large Pharmaceutical Companies,« American Journal of Medical Research, pp. 141–151, 2016.
[11] H. Baars, P. Weber and A. Tank, »Institutionalizing Analytic Data Sharing in SME Ecosystems - A Role-Based Perspective,« in HICSS, 2022.
[12] P. T. Ng, »Learning in an era of uncertainty in Singapore: diversity, lifelong learning, inspiration and paradigm shift,« Educational Research for Policy and Practice, 22 May 2023.
Letzte Änderung: